Gaspard Beugnot
Machine Learning & Optimization for quantum chip design
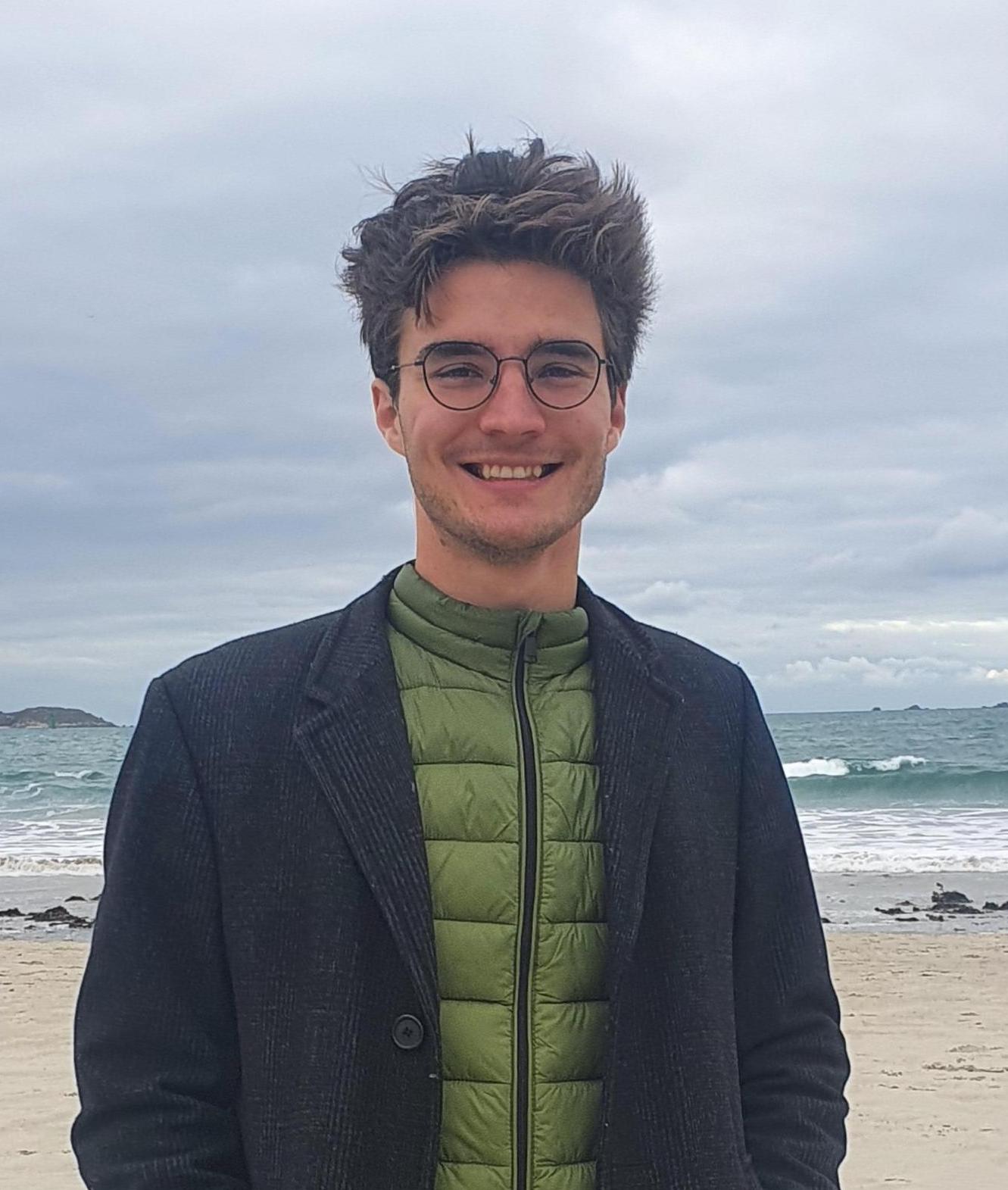
I’m currently a research scientist in Machine Learning and Optimization at Alice & Bob, to help design cat qubits to achieve fault-tolerant quantum computing. Specifically, I work on simulating quantum systems, chips calibration and state measurements with a mix of learning, optimization, optimal control and experiment design. The intersection between quantum physics and ML&Opt is fascinating!
Before that I obtained a PhD from ENS and Inria, under the supervision of Julien Mairal and Alessandro Rudi. My research focused on theoretical properties of kernel methods in all sorts of flavour. I’m also interested in scientific computing in general, and an user of Julia, Jax, and various flavours of functional programing. Find the manuscript here!
I’m always opened to academic collaborations. Don’t hesitate to reach out!
And before that, I graduated from Ecole Polytechnique (X2016) and got a master from École Normale Supérieure in Mathematics, Vision and Machine Learning in 2020 (Master MVA).
news
Jul 22, 2024 | I will present at ISMP2024 in Montreal! |
---|---|
Apr 5, 2024 | I defended my PhD thesis! Optimization, Generalization and Non-Convex Optimization with kernel methods 🥳 |
Mar 1, 2024 | I joined Alice & Bob as a Machine Learning Research Scientist! |
Sep 28, 2023 | Our GloptiNets paper was awarded a spotlight presentation at NeurIPS 2023 🎉 See you there! |
Jul 3, 2023 | Check my latest preprint on GloptiNets! An algorithm which provides certificates to non-convex optimization problems. |
Jun 23, 2023 | I stumbled upon Google’s Foobar challenge. I doubt it’ll actually get me an interview, but it’s definitely a sneaky way to trap you into spending a ton of time on some tricky brain teasers. |
Apr 1, 2023 | I’m working on polynomial optimization (with certificates!) – if you have real-life problems involving those, reach me out! |
May 20, 2022 | Our paper on the influence of the learning on the generalization was accepted at COLT22! See you there! |
Apr 15, 2022 | The learning rate impacts the generalization when training neural network. Did you know it can occur in convex settings too? Check our last preprint! |
Sep 28, 2021 | My paper on the proximal point method for concordant loss function was awarded a Spotlight award at NeurIPS 2021! |
latest posts
Mar 30, 2023 | From Python to Julia |
---|